Data Virtualization for Business Intelligence Systems is available from the publisher and from
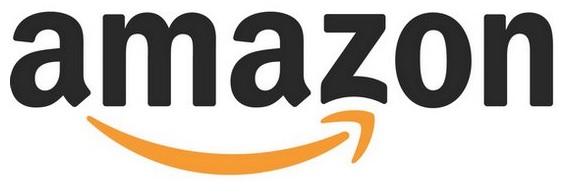
Introduction
Data virtualization can make business intelligence systems dramatically simpler, cheaper, and, most importantly, more agile. Learn what data virtualization is and how and why it should be used. The book explains how data virtualization works, the impact it has on business intelligence systems, which techniques are to used under the hood to optimize access to various data sources, and how these products can be applied in different projects. You’ll learn the differences between this new form of data integration and more well-known forms, such as ETL and replication and gain a clear understanding of how data virtualization really works.
Data Virtualization for Business Intelligence Systems outlines the advantages and disadvantages of data virtualization and illustrates how this revolutionary technology should be applied in data warehouse environments. The book also contains tips and do’s and don’ts on how to adopt data virtualization, provides guidelines on how to use it efficiently and effectively. It also describes the relationship between data virtualization and related topics, such as master data management, data governance, information management, and service oriented architectures, giving you a big-picture understanding as well as all the practical know-how you need to virtualize your data.
Features:
-
First independent book on data virtualization that explains how data virtualization technology works in a product-independent way.
-
Illustrates concepts using numerous examples, screenshots (developed with commercially available products), and diagrams.
-
Shows you how to solve common data integration challenges, such as data quality, unstructured data sources, big data, inconsistent data sources, and overall performance by following practical guidelines on using data virtualization.
-
Explains the various application areas of data virtualization, such as virtual data marts, extended data warehouse,
-
Presents the big picture of data virtualization and its relationship with data governance, data quality, and information management.
Downloading the Sample Database
This sample database used throughout the book is a subset of the one designed by Roland Bouman and Jos van Dongen for their book Pentaho Solutions. If you're interested in loading this sample database, you can download the full original database via the following link. The only file you have to download is the one called SQL scripts (wcm.sql). This file is developed for the MySQL database server. Note that only seven of the tables form this database are used in the book; see Section 1.14.
Table of Contents
1. Introduction to Data Virtualization
-
1.1 Introduction
-
1.2 The World of Business Intelligence is Changing
-
1.3 Introduction to Virtualization
-
1.4 What is Data Virtualization?
-
1.5 Data Virtualization and Related Concepts
-
1.6 Definition of Data Virtualization
-
1.7 Technical Advantages of Data Virtualization
-
1.8 Different Implementations of Data Virtualization
-
1.9 Overview of Data Virtualization Servers
-
1.10 Open versus Closed Data Virtualization Servers
-
1.11 Other Forms of Data Integration
-
1.12 The Modules of a Data Virtualization Server
-
1.13 The History of Data Virtualization
-
1.14 The Sample Database: World Class Movies
-
1.15 Structure of the Book
2. Business Intelligence and Data Warehousing
-
2.1 Introduction
-
2.2 What is Business Intelligence?
-
2.3 Management Levels and Decision-Making
-
2.4 Business Intelligence Systems
-
2.5 The Data Stores of a Business Intelligence System
-
2.6 Normalized Schemas, Star Schemas, and Snowflake Schemas
-
2.7 Data Transformation with ETL, ELT, and Replication
-
2.8 Overview of Business Intelligence Architectures
-
2.9 New Forms of Reporting and Analytics
-
2.10 Disadvantages of Classic Business Intelligence Systems
-
2.11 Summary
3. Data Virtualization Server: The Building Blocks
-
3.1 Introduction
-
3.2 The High-Level Architecture of a Data Virtualization Server
-
3.3 Importing Source Tables and Defining Wrappers
-
3.4 Defining Virtual Tables and Mappings
-
3.5 Examples of Virtual Tables and Mappings
-
3.6 Virtual Tables and Data Modeling
-
3.7 Nesting Virtual Tables and Shared Specifications
-
3.8 Importing Non-Relational Data
-
3.9 Publishing Virtual Tables
-
3.10 The Internal Data Model
-
3.11 Updatable Virtual Tables and Transaction Management
4. Data Virtualization Server: Management and Security
-
4.1 Introduction
-
4.2 Impact and Lineage Analysis
-
4.3 Synchronization of Source Tables, Wrapper Tables, and Virtual Tables
-
4.4 Security of Data: Authentication and Authorization
-
4.5 Monitoring, Management, and Administration
5. Data Virtualization Server: Caching of Virtual Tables
-
5.1 Introduction
-
5.2 The Cache of a Virtual Table
-
5.3 When to Use Caching?
-
5.4 Caches versus Data Marts
-
5.5 Where is the Cache Kept?
-
5.6 Refreshing Caches
-
5.7 Full Refreshing, Incremental Refreshing, and Live Refreshing
-
5.8 Online Refreshing and Offline Refreshing
-
5.9 Cache Replication
6. Data Virtualization Server: Query Optimization Techniques
-
6.1 Introduction
-
6.2 A Refresher on Query Optimization
-
6.3 The Ten Stages of Query Processing by a Data Virtualization Server
-
6.4 The Intelligence Level of the Data Stores
-
6.5 Optimization through Query Substitution
-
6.6 Optimization through Pushdown
-
6.7 Optimization through Query Expansion (Query Injection)
-
6.8 Optimization through Ship Joins
-
6.9 Optimization through Sort-Merge Joins
-
6.10 Optimization by Caching
-
6.11 Optimization and Statistical Data
-
6.12 Optimization through Hints
-
6.13 Optimization through SQL Override
-
6.14 Explaining the Processing Strategy
7. Deploying Data Virtualization in Business Intelligence Systems
-
7.1 Introduction
-
7.2 A Business Intelligence System based on Data Virtualization
-
7.3 Advantages of Deploying Data Virtualization
-
7.4 Disadvantages of Deploying Data Virtualization
-
7.5 Strategies for Adopting Data Virtualization
-
7.6 Application Areas of Data Virtualization
-
7.7 Myths on Data Virtualization
8. Design Guidelines for Data Virtualization
-
8.1 Introduction
-
8.2 Incorrect Data and Data Quality
-
8.3 Complex and Irregular Data Structures
-
8.4 Implementing Transformations in Wrappers or Mappings
-
8.5 Analyzing Incorrect Data
-
8.6 Different Users and Different Definitions
-
8.7 Time Inconsistency of Data
-
8.8 Data Stores and Data Transmission
-
8.9 Retrieving Data from Production Systems
-
8.10 Joining Historical and Operational Data
-
8.11 Dealing with Organizational Changes
-
8.12 Archiving Data
9. Data Virtualization and SOA
-
9.1 Introduction
-
9.2 SOA in a Nutshell
-
9.3 Basic Services, Composite Services, Business Process Services, and Data Services
-
9.4 Developing Data Services with a Data Virtualization Server
-
9.5 Developing Composite Services with a Data Virtualization Server
-
9.6 Services and the Internal Data Model
10. Data Virtualization and Master Data Management
-
10.1 Introduction
-
10.2 Data is a Critical Asset for Every Organization
-
10.3 The Need for a 360° View of Business Objects
-
10.4 What is Master Data?
-
10.5 What is Master Data Management?
-
10.6 A Master Data Management System
-
10.7 Master Data Management for Integrating Data
-
10.8 Integrating Master Data Management and Data Virtualization
11. Data Virtualization, Information Management, and Data Governance
-
11.1 Introduction
-
11.2 Impact of Data Virtualization on Information Modeling and Database Design
-
11.3 Impact of Data Virtualization on Data Profiling
-
11.4 Impact of Data Virtualization on Data Cleansing
-
11.5 Impact of Data Virtualization on Data Governance
12. The Data Delivery Platform – A New Architecture for Business Intelligence Systems
-
12.1 Introduction
-
12.2 The Data Delivery Platform in a Nutshell
-
12.3 The Definition of the Data Delivery Platform
-
12.4 The Data Delivery Platform and Other Business Intelligence Architectures
-
12.5 The Requirements of the Data Delivery Platform
-
12.6 The DDP versus Data Virtualization
-
12.7 Explanation of the Name
-
12.8 A Personal Note
13. The Future of Data Virtualization
-
13.1 Introduction
-
13.2 The Future of Data Virtualization According to Rick F. van der Lans
-
13.3 The Future of Data Virtualization According to David Besemer, CTO of Composite Software
-
13.4 The Future of Data Virtualization According to Alberto Pan, CTO of Denodo Technologies
-
13.5 The Future of Data Virtualization According to James Markarian, CTO of Informatica Corporation
|